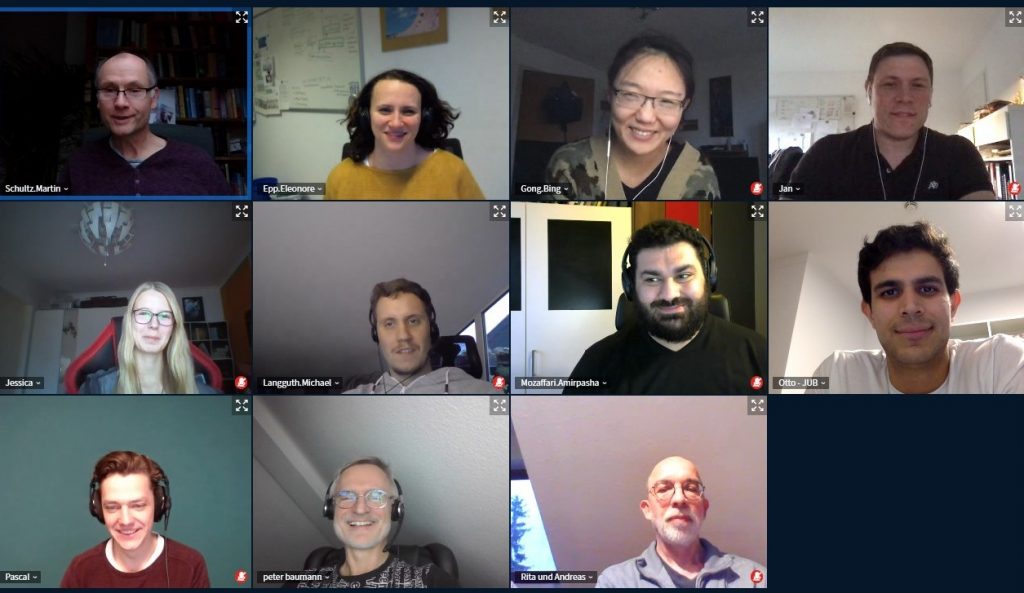
In this project meeting, which again took place virtually, the project progress of the past months was discussed. There was some pleasing progress to report here, although the interdisciplinary collaboration proved more difficult than hoped in some cases due to the pandemic situation.
- The University of Bonn has carried out an analysis of how large-scale weather data affect the occurrence of precipitation at the Münster and Osnabrück measuring stations. For this purpose, a so-called logistic regression was used. Depending on the season, this approach results in a significant improvement compared to a purely local forecast.
- The DWD tested different approaches for a regression with a generalised linear model and, in particular, investigated to what extent it makes a difference whether the input variables are selected separately for each measuring station or jointly for all of them. In fact, even with a generalised approach, similar good results can be obtained for the estimation of precipitation as with the separate procedure. However, the yes-no decision of whether it will rain or not becomes less accurate at some stations.
- Jacobs University used Jupyter notebooks to demonstrate how queries from the Rasdaman database can be integrated into data analysis and machine learning workflows. Furthermore, Jülich was supported to join the data federation in the Earth Server data cube.
- Forschungszentrum Jülich reported progress in the development of machine learning workflows, much of which has now been parallelized, greatly increasing the throughput of data and computations. Data management for the huge amount of weather data is now largely consolidated; in the main, radar data still needs to be finalized for processing.
- The University of Osnabrück successfully applied neural networks to learn the relation of next-day precipitation amounts to current weather data at a small set of measurement stations. The neural networks outperformed classical regression. Implementing the machine learning workflow on the Jülich supercomputer presented some challenges due to the need of an efficient and flexible data handling tool, which would work on the vast amount of raw data that is available in the project.